The business environment is dynamic, and factors influencing it can change rapidly. A predictive model built on historical data may become less effective as the business landscape evolves. Refreshing the model allows it to adapt to new trends, emerging patterns, and shifts in customer behavior.
Over time, the data used to train predictive models may experience drift. Changes in customer preferences, market conditions, or external factors can lead to a disconnect between the training data and the current reality. Addressing data drift and model drift is essential to maintain the model’s accuracy.
The field of data science is constantly evolving, with new algorithms, techniques, and technologies emerging regularly. A model that was state-of-the-art a few years ago may now benefit from advancements in machine learning. Refreshing the model allows integration of the latest methodologies to improve its predictive capabilities.
Feedback Loops and Continuous Learning
Predictive models can benefit from continuous learning through feedback loops. Updating the model based on real-world outcomes and incorporating new data enhances its ability to make accurate predictions. A refresh ensures that the model remains adaptive and responsive to changing conditions.
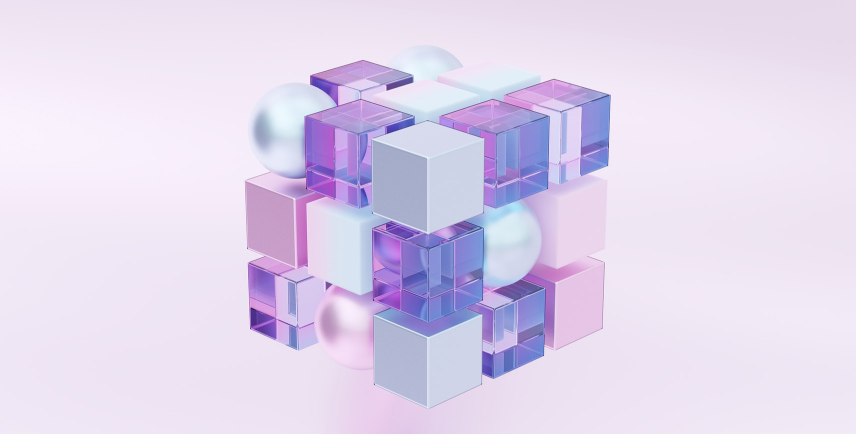
Enhanced Feature Engineering
As understanding of the business domain deepens, opportunities for more nuanced and effective feature engineering may arise. A model refresh provides the chance to incorporate new features or refine existing ones, allowing for a more comprehensive representation of the underlying patterns in the data.
Regulatory Compliance and Ethical Considerations
Changes in regulations and ethical standards may necessitate a refresh of predictive models. Ensuring compliance with evolving legal frameworks and ethical guidelines is crucial, especially in industries where data privacy and ethical considerations play a significant role.
Refreshing a predictive model provides an opportunity to enhance its interpretability. Models that are more interpretable are not only easier to understand but also instill greater confidence in stakeholders, making them more likely to be adopted and trusted in decision-making processes.